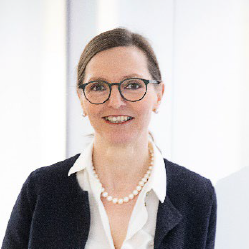
Ruth Otto
Professor for Robotics and Industrial Automation
University of Applied Sciences Munich
Prof. Ruth Otto has over 20 years of experience in the industry, including ten years in a leadership role in the development department of KUKA Roboter GmbH. Her expertise spans a wide range of robotics development, from fundamental research to advanced applications with a forward-looking perspective.
In 2011, she became an independent project consultant for robotics and automation, focusing on assistive robotics and human-robot collaboration, integrating imaging and force-moment sensing technologies. Since March 2020, Prof. Otto has been a Professor of Robotics and Automation at the University of Applied Sciences in Munich. She maintains strong industry collaborations with companies such as BMW, Universal Robots, and KUKA to prepare students for careers in industry and applied research.
AI-Driven Sensor-Based Robotics for Sustainable and Flexible Manufacturing
Presentation Abstract:
Sensor-based robotic applications, such as vision- or force-torque-controlled systems, are essential for meeting current and future industrial demands. Manufacturing processes must become more flexible and adaptive while simultaneously reducing energy consumption. The growing need for customized production on demand, efficient disassembly of used or defective components for recycling, and user-friendly robot operation and programming requires a seamless integration of data from humans, robots, sensors, and synthetic sources. The intelligent combination of these elements enables highly flexible and efficient process automation.
In the BMWK-funded project GreenBotAI, artificial intelligence (AI) is leveraged to significantly reduce setup times for vision- and force-torque-controlled applications. A key objective is the transition from static applications to adaptive, real-time on-the-fly processes. Additionally, lightweight AI models are employed to optimize energy efficiency further. A digital twin is utilized to generate synthetic data, enhancing simulation, model training, and validation of robotic tasks for greater efficiency and adaptability.
A planned follow-up project will focus on the enrichment of digital design data with synthetic and sensor-derived information from images and force feedback. This will support automated disassembly processes for the circular economy. Moreover, innovative strategies for adaptive path planning and situation-aware human-robot collaboration will be developed to enhance efficiency and sustainability in industrial automation.